15 Application: Meta-analysis using (some of) the METARET data
Sometimes, you have more than one dataset and you want to make inferences about how similar or dissimular these datasets are, or how much you can learn from the data as a whole. This is where you want to do a meta-analysis, and Bayesian techniques are particularly well-suited to these, for exactly the same reason that they handle hierarchical models well. While the hierarchical models presented up to this point has two levels of parameters (i.e. population-level and participant-level), we will now have three or more levels, as there could be meaningful variation at the population level, between experiments, and between participants within experiments. Quantifying this variation is useful, and some small additions to our understanding of hierarchical models will help us understand this.
Hopefully you will see that adding this additional level of variation is not much of a leap forward as far as hierarchical models go. We now simply have (i) parameters describing the distribution between experiments. These feed into (ii) parameters describing the distribution within experiments, and (iii) participant-specific parameters.
I suspect and hope that this kind of analysis is going to become very common in Experimental Economics. This is because we have had a long history of good data-sharing norms, and it is therefore not too difficult to obtain data from published experiments. Furthermore, as experimental economists often design new experiments by using older experiments as a baseline, there are a lot of opportunities for the same (or similar enough) designs to show up a lot more than once.
15.1 Data
Here we will be using a subset of the METARET datset, which is a collection of data on risk elicitation tasks.76 I will focus just on the subset of this dataset corresponding to the Holt and Laury (2002) risk elicitation task. Table 15.1 summarizes this slice of the data. as you can see, there are a lot of experiments, and a lot of participants!
D<-"Code/METARET/Data/data.csv" |>
read.csv() |>
filter(task=="HL")|>
mutate(exptID = bibkey |> as.factor())
exptList<-D |>
group_by(exptID) |>
summarize(
experiment = bibkey[1],
id = exptID[1] |> as.numeric(),
participants = n()
)
exptList |>
dplyr::select(
experiment,participants
) |>
kbl(caption = paste("The ",dim(exptList)[1],"experiments using the Holt and Laury (2002) task in the METARET dataset")) |>
kable_classic(full_width=FALSE)
experiment | participants |
---|---|
Abdellaoui2011 | 36 |
Andersen2010 | 89 |
Barrera2012 | 98 |
Bauernschuster2010 | 174 |
Bellemare2010 | 84 |
Branas2011 | 145 |
Carlsson2009 | 213 |
Casari2009 | 78 |
Chakravarty2011 | 37 |
Charness2019 | 157 |
Chen2013 | 72 |
Cobo-Reyes2012 | 76 |
Crosetto2016 | 73 |
Dave2010 | 802 |
Deck2012a | 47 |
Dickinson2009 | 126 |
Drichoutis2012 | 57 |
Duersch2012 | 200 |
Eckel2006 | 198 |
Fiedler2012 | 29 |
Fiore2009 | 40 |
Frey2017 | 1331 |
Gloeckner2012 | 159 |
Gloeckner2012a | 38 |
Grijalva2011 | 77 |
Harrison2005 | 152 |
Harrison2007b | 18 |
Harrison2008 | 100 |
harrison2012b | 90 |
Holt2002 | 199 |
Holzmeister2019 | 198 |
Jacquemet2008 | 87 |
jamison2008 | 130 |
Lange2007 | 121 |
Lange2007a | 172 |
Levy-Garboua2012 | 54 |
Lusk2005 | 47 |
Masclet2009 | 79 |
Mueller2012 | 116 |
Nieken2012 | 287 |
Pogrebna2011 | 57 |
Ponti2009 | 33 |
Rosaz2012 | 112 |
Rosaz2012a | 279 |
Ryvkin2011 | 42 |
Schram2011 | 137 |
Shafran2010 | 64 |
Slonim2010 | 116 |
Sloof2010 | 86 |
Szrek2012 | 198 |
Wakolbinger2009 | 131 |
Yechiam2012 | 11 |
Table 15.2 shows you a random sample of the data we will be using. The variable bibkey
will identify the experiment. Our outcome of interest will be the variable r
, wich is an estimate of the coefficient of relative risk aversion. However those of you familiar with Holt and Laury (2002) will know that this task only gives you an interval range for \(r\). This interval is a function of the variable choice
, which is the switch-point observed for a participant in the task. In corresponding with Paolo Crosetto, I found out that the r
variable was uniformly assigned within this range (you can also see this in the METARET replication files). We can see that these intervals are exactly the same by looking at the empirical cumulative density function of the r
variable, which I show in Figure 15.1:
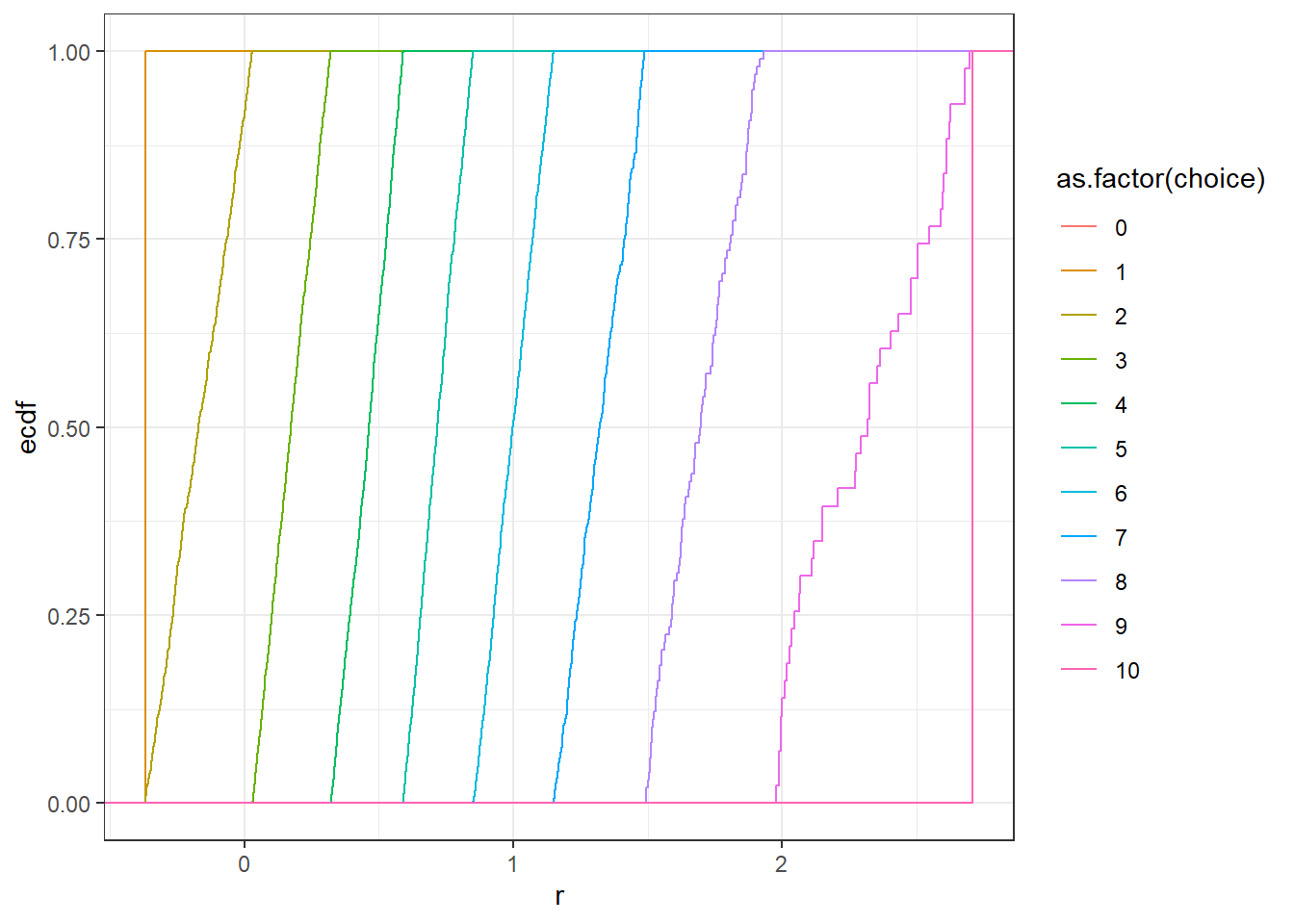
Figure 15.1: Empirical cumulative density functions of \(r\), broken down by choice in the Holt and Laury (2002) task
For simplicity we will begin by treating r
as if it is the exact value of \(r\) for this participant, but then we will relax this assumption so that it is interval-valued.
set.seed(42)
D |>
sample_n(20) |>
dplyr::select(bibkey,choice,r,task) |>
kbl(caption = "A random sample of the METARET Holt and Laury (2002) data.") |>
kable_classic(full_width=FALSE)
bibkey | choice | r | task |
---|---|---|---|
harrison2012b | 5 | 0.7974468 | HL |
Duersch2012 | 6 | 1.1191757 | HL |
Lange2007a | 5 | 0.6087734 | HL |
Grijalva2011 | 6 | 1.1030496 | HL |
Szrek2012 | 5 | 0.6409813 | HL |
Jacquemet2008 | 6 | 0.9068044 | HL |
Frey2017 | 3 | 0.0869766 | HL |
Shafran2010 | 7 | 1.3751412 | HL |
Gloeckner2012 | 2 | -0.2327934 | HL |
Grijalva2011 | 8 | 1.4956184 | HL |
Andersen2010 | 4 | 0.5473514 | HL |
Branas2011 | 5 | 0.8301501 | HL |
Bauernschuster2010 | 4 | 0.4462529 | HL |
Holzmeister2019 | 3 | 0.0638232 | HL |
Casari2009 | 5 | 0.7920361 | HL |
Carlsson2009 | 1 | -0.3700000 | HL |
Frey2017 | 2 | -0.2673258 | HL |
Frey2017 | 5 | 0.7556473 | HL |
Duersch2012 | 4 | 0.3521928 | HL |
Wakolbinger2009 | 7 | 1.3328647 | HL |
15.2 A basic model
To begin with, assume that the r
in the dataset is measured perfectly, and so we do not have to worry about it really being an interval. Let \(r_{i,e}\) be the risk-aversion value measured for participant \(i\) in experiment \(e\). Within each experiment \(e\), assume that \(r_{i,e}\sim N(\mu_e,\sigma^2)\), where \(\mu_e\) is an experiment-specific mean of \(r_{i,e}\) and \(\sigma\) is common to all experiments (we will relax this later). Between experiments, we will assume that the experiment means \(\mu_i\)s are normally distributed, \(\mu_i\sim N(\bar\mu,\bar\sigma^2)\). That is, we have between-experiment variation measured by \(\bar\sigma\), and within-experiment variation measured by \(\sigma\).
As we might be interested in the relative importance of these sources of variation, it is useful to decompose the total variance of an observation by looking at the ratio of between-experiment variance to within-experiment variance, which is:
\[ \rho = \frac{\bar\sigma^2}{\bar\sigma^2+\sigma^2} \]
That is if \(\rho\) is close to zero, then \(\bar\sigma^2\) is relatively small. This will mean that there is not much difference in the means of \(r_{e,i}\) between experiments. On the other hand, if \(\rho\) is close to one, then most of the variation in \(r_{e,i}\) is attributable to variation between experiments. In this case, it would be hard to predict the mean of \(r_{e,i}\) in a new experiment.
Here is my formal statement of the model’s structure and its priors:
\[ \begin{aligned} \text{Participant-level variation: }r_{i,e}&\sim N(\mu_e,\sigma^2)\\ \text{Experiment-level variation: }\mu_e&\sim N(\bar\mu,\bar\sigma^2)\\ \text{priors: } \bar\mu&\sim N(0,10)\\ \bar\sigma&\sim\mathrm{Cauchy}^+(0,10)\\ \sigma&\sim \mathrm{Cauchy}^+(0,10) \end{aligned} \]
And here is the Stan program I wrote to estimate it. Note that we are augmenting the data by the \(\mu_i\)s. Therefore we will get shrinkage estimates of these from the estimation process.
// 2level.stan
// a 2-level hierarchical model for aggregating risk preferences
data {
int<lower=0> N;
vector[N] r;
int nexpt;
int exptID[N];
vector[2] prior_MU;
real prior_sigma_expt;
real prior_sigma_i;
}
parameters {
real MU;
real<lower=0> sigma_expt;
real<lower=0> sigma_i;
vector[nexpt] mu_expt;
}
model {
// prior
target += normal_lpdf(MU | prior_MU[1],prior_MU[2]);
target += cauchy_lpdf(sigma_expt | 0.0,prior_sigma_expt);
target += cauchy_lpdf(sigma_i | 0.0,prior_sigma_i);
// hierarchical structure
target+= normal_lpdf(mu_expt | MU,sigma_expt);
// likelihood
target += normal_lpdf(r | mu_expt[exptID], sigma_i);
}
generated quantities {
real rho = pow(sigma_expt,2.0)/(pow(sigma_expt,2.0)+pow(sigma_i,2.0));
}
Table 15.3 shows the estimates from this model. In particular, note the estimate for \(\rho\), which is much closer to zero than one. This suggests that within-experiment variation in \(r_{e,i}\) is larger than between-experiment variation in its means.
Fit<-summary(
"Code/METARET/Fit.rds" |>
readRDS()
)$summary |>
data.frame()|>
rownames_to_column(var="parameter") |>
mutate(
exptID = parse_number(parameter)
)
Fit |>
filter(is.na(exptID)) |>
dplyr::select(-exptID) |>
kbl(digits=3,caption = "Parameter estimates from the basic model") |>
kable_classic(full_width=FALSE)
parameter | mean | se_mean | sd | X2.5. | X25. | X50. | X75. | X97.5. | n_eff | Rhat |
---|---|---|---|---|---|---|---|---|---|---|
MU | 0.584 | 0.00 | 0.030 | 0.524 | 0.564 | 0.584 | 0.604 | 0.643 | 5618.174 | 1.000 |
sigma_expt | 0.207 | 0.00 | 0.025 | 0.165 | 0.189 | 0.206 | 0.223 | 0.261 | 4218.509 | 1.000 |
sigma_i | 0.550 | 0.00 | 0.005 | 0.541 | 0.547 | 0.550 | 0.553 | 0.559 | 7046.200 | 1.000 |
rho | 0.125 | 0.00 | 0.026 | 0.082 | 0.106 | 0.122 | 0.141 | 0.184 | 4256.043 | 1.000 |
lp__ | -6203.000 | 0.13 | 5.223 | -6214.325 | -6206.201 | -6202.631 | -6199.302 | -6193.737 | 1611.583 | 1.003 |
15.3 But the data are really interval-valued!
Now we will take the interval-valued property of Holt and Laury (2002) data more seriously. Once we code up the bounds (you can see how I do that at the end of this chapter), it is really easy to augment our data again by the unobserved (i.e. latent) \(r_{i,i}^*\), which we know will be somewhere between a lower bound of \(\underline r_{e,i}\), and an upper bound of \(\overline r_{e,i}\). Here is the modification to the model statement we need to make:
\[ \begin{aligned} \text{Participant-level variation: }r_{i,e}^*&\sim \mathrm{Truncated\ Normal}(\mu_e,\sigma^2,(\underline r_{i,e},\overline r_{i,e}))\\ \text{Experiment-level variation: }\mu_e&\sim N(\bar\mu,\bar\sigma^2)\\ \text{priors: } \bar\mu&\sim N(0,10)\\ \bar\sigma&\sim\mathrm{Cauchy}^+(0,10)\\ \sigma&\sim \mathrm{Cauchy}^+(0,10) \end{aligned} \]
And here is the Stan program that estimates it. Note now that r
is a parameter, rather than data.
// 2level_interval.stan
// a 2-level hierarchical model for aggregating risk preferences
// treating choices as revealing intervals
data {
int<lower=0> N; // number of observations
vector[N] rlb; // lower bound for r
vector[N] rub; // upper bound of r
int nexpt; // number of experiments
int exptID[N]; // experiment id
vector[2] prior_MU; // mean of r in population
real prior_sigma_expt; // sd of r at experiment level
real prior_sigma_i; // sd of r at individual level
}
parameters {
real MU;
real<lower=0> sigma_expt;
real<lower=0> sigma_i;
vector[nexpt] mu_expt;
vector<lower=rlb,upper=rub>[N] r;
}
model {
// prior
target += normal_lpdf(MU | prior_MU[1],prior_MU[2]);
target += cauchy_lpdf(sigma_expt | 0.0,prior_sigma_expt);
target += cauchy_lpdf(sigma_i | 0.0,prior_sigma_i);
// hierarchical structure
target+= normal_lpdf(mu_expt | MU,sigma_expt);
// likelihood
target += normal_lpdf(r | mu_expt[exptID], sigma_i);
}
generated quantities {
real rho = pow(sigma_expt,2.0)/(pow(sigma_expt,2.0)+pow(sigma_i,2.0));
}
Table 15.4 shows the estimates from this model. These are not substantially different from those in Table 15.3, but we didn’t know that until estimating this model.
Fit_interval<-summary(
"Code/METARET/Fit_interval.rds" |>
readRDS()
)$summary |>
data.frame()|>
rownames_to_column(var="parameter") |>
mutate(
exptID = parse_number(parameter)
)
Fit_interval |>
filter(is.na(exptID)) |>
dplyr::select(-exptID) |>
kbl(digits=3,caption = "Parameter estimates from the interval-valued model") |>
kable_classic(full_width=FALSE)
parameter | mean | se_mean | sd | X2.5. | X25. | X50. | X75. | X97.5. | n_eff | Rhat |
---|---|---|---|---|---|---|---|---|---|---|
MU | 0.567 | 0.001 | 0.034 | 0.501 | 0.544 | 0.568 | 0.591 | 0.632 | 4096.224 | 1.000 |
sigma_expt | 0.230 | 0.000 | 0.027 | 0.183 | 0.211 | 0.228 | 0.247 | 0.290 | 3068.388 | 1.000 |
sigma_i | 0.590 | 0.000 | 0.005 | 0.580 | 0.587 | 0.590 | 0.594 | 0.601 | 5038.230 | 1.000 |
rho | 0.133 | 0.000 | 0.027 | 0.087 | 0.113 | 0.130 | 0.149 | 0.193 | 3050.136 | 1.000 |
lp__ | -30049.240 | 2.011 | 73.787 | -30196.315 | -30099.434 | -30049.547 | -29998.176 | -29905.889 | 1346.326 | 1.001 |
15.4 Heterogeneous standard deviations
Of course, these experiments could be different not just because they have different means of risk-aversion, but also because they have different standard deviations of risk-aversion. In order to investigate how and whether this affects our conclusions, here is a model that assumes a hierarchical structure for \(\sigma_e\) as well as \(\mu_e\):
\[ \begin{aligned} \text{Participant-level variation: }r_{i,e}^*&\sim \mathrm{Truncated\ Normal}(\mu_e,\sigma^2_e,(\underline r_{i,e},\overline r_{i,e}))\\ \text{Experiment-level variation: }\mu_e&\sim N(\bar\mu,\bar\sigma^2)\\ \log \sigma_e &\sim N(M,S^2)\\ \text{priors: } \bar\mu&\sim N(0,10)\\ \bar\sigma&\sim\mathrm{Cauchy}^+(0,10)\\ M&\sim N(0,10)\\ S&\sim \mathrm{Cauchy}^+(0,10) \end{aligned} \]
And here is the the Stan file that implements it. Note that we are augmenting the data by \(\sigma_e\) and \(\mu_e\) now. Also, since we don’t have one \(\rho\) anymore, I am calculating an average \(\rho\) in the generated quantities
block using Monte Carlo integration.
// 2level_interval_sd.stan
// a 2-level hierarchical model for aggregating risk preferences
// treating choices as revealing intervals
data {
int<lower=0> N; // number of observations
vector[N] rlb; // lower bound for r
vector[N] rub; // upper bound of r
int nexpt; // number of experiments
int exptID[N]; // experiment id
vector[2] prior_MU; // mean of r in population
real prior_sigma_expt; // sd of r at experiment level
vector[2] prior_sigma_i_M; // m and sd of log-median sigma_i
real<lower=0> prior_sigma_i_SD; // sd for sigma_i
int nsim;
}
parameters {
real MU;
real<lower=0> sigma_expt;
real sigma_i_M;
real<lower=0> sigma_i_SD;
// augmentted data
vector<lower=0>[nexpt]sigma_i;
vector[nexpt] mu_expt;
vector<lower=rlb,upper=rub>[N] r;
}
model {
// prior
target += normal_lpdf(MU | prior_MU[1],prior_MU[2]);
target += cauchy_lpdf(sigma_expt | 0.0,prior_sigma_expt);
target += normal_lpdf(sigma_i_M | prior_sigma_i_M[1],prior_sigma_i_M[2]);
target += cauchy_lpdf(sigma_i_SD | 0.0, prior_sigma_i_SD);
// hierarchical structure
target+= normal_lpdf(mu_expt | MU,sigma_expt);
target+= lognormal_lpdf(sigma_i | sigma_i_M, sigma_i_SD);
// likelihood
target += normal_lpdf(r | mu_expt[exptID], sigma_i[exptID]);
}
generated quantities {
real rho;
{
vector[nsim] sim_sigma_i =exp(sigma_i_M+sigma_i_SD*to_vector(normal_rng(rep_vector(0.0,nsim),rep_vector(1.0,nsim))));
rho = mean(pow(sigma_expt,2.0)/(pow(sigma_expt,2.0)+pow(sim_sigma_i,2.0)));
}
}
Table 15.5 shows the estimates of the non-augmented parameters of the model. We don’t see much of a difference in what we can compare to the previous model. However note that sigma_i_sd
is substantially away from zero, indicating a good amount of variation in the experiment-specific standard deviations.
Fit_interval_sd<-summary(
"Code/METARET/Fit_interval_sd.rds" |>
readRDS()
)$summary |>
data.frame()|>
rownames_to_column(var="parameter") |>
mutate(
exptID = parse_number(parameter)
)
Fit_interval_sd |>
filter(is.na(exptID)) |>
dplyr::select(-exptID) |>
kbl(digits=3,caption = "Parameter estimates from the model assuming heterogeneous within-experiment standard deviations") |>
kable_classic(full_width=FALSE)
parameter | mean | se_mean | sd | X2.5. | X25. | X50. | X75. | X97.5. | n_eff | Rhat |
---|---|---|---|---|---|---|---|---|---|---|
MU | 0.550 | 0.000 | 0.028 | 0.496 | 0.531 | 0.550 | 0.570 | 0.606 | 3834.973 | 1.000 |
sigma_expt | 0.186 | 0.000 | 0.024 | 0.144 | 0.169 | 0.184 | 0.201 | 0.239 | 2679.907 | 1.002 |
sigma_i_M | -0.521 | 0.001 | 0.044 | -0.608 | -0.551 | -0.521 | -0.490 | -0.436 | 4478.523 | 1.000 |
sigma_i_SD | 0.316 | 0.001 | 0.037 | 0.253 | 0.290 | 0.313 | 0.339 | 0.396 | 3509.713 | 1.000 |
rho | 0.104 | 0.000 | 0.025 | 0.064 | 0.086 | 0.101 | 0.118 | 0.159 | 2969.940 | 1.001 |
lp__ | -29589.388 | 2.016 | 73.080 | -29738.524 | -29637.172 | -29587.289 | -29540.121 | -29451.549 | 1314.469 | 1.001 |
Now that we have two sets of augmented parameters, \(\mu_e\) and \(\sigma_e\), we can visualize their relationship in a plot, which I do in Figure 15.2. These are the “shrinkage” estimates of these parameters, in that they are informed both by the values of \(r_{i,e}\) within their respective experiments, and also by the population distributions that are jointly estimated alongside them.
d<-Fit_interval_sd |>
filter(!is.na(exptID)) |>
mutate(parameter = gsub("[^a-zA-Z]", "", parameter)) |>
pivot_wider(
id_cols = exptID,
names_from = "parameter",
values_from = c("mean","X25.","X75.")
) |>
mutate(id = exptID |> as.numeric()) |>
full_join(
exptList,
by = "id"
)
(
ggplot(d,aes(x=mean_muexpt,y=mean_sigmai,label=experiment))
#+geom_text(size=2)
+geom_point(aes(size=participants))
+geom_errorbar(aes(xmin = X25._muexpt,xmax=X75._muexpt),alpha=0.3)
+geom_errorbar(aes(ymin = X25._sigmai,ymax=X75._sigmai),alpha=0.3)
+xlab(expression(mu[e]~": mean of risk-aversion within experiemnt"))+ylab(expression(sigma[e]~": standard deviation of risk-aversion within experiment"))
+theme_bw()
)
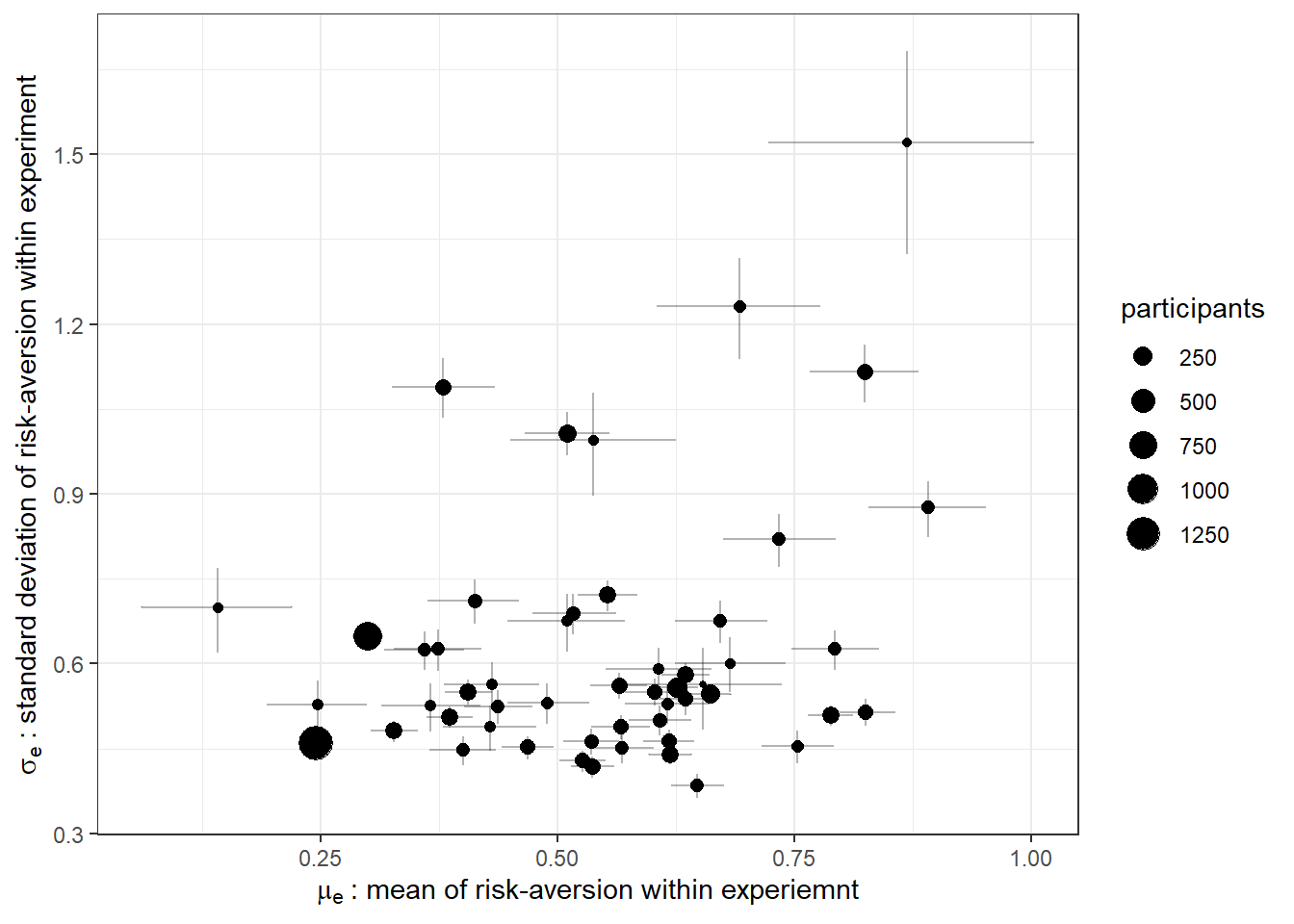
Figure 15.2: Experiment-level estimates of mean and standard deviation of risk-aversion. Error bars show 50% Bayesian credible regions
15.5 Student-\(t\) distributions, because why not?
One assumption we have maintained here is that the experiment- and participant-level distributions are both normals. While this is a great place to start, but if we suspect that this is a bad assumption then we might want to take a more flexible model to our data. A simple one-parameter relaxation of the normal distribution is Student’s \(t\) distribution. The new “degrees of freedom” parameter \(\nu>0\) measures how closely this distribution matches the normal: as \(\nu\to\infty\) the \(t\) approaches the normal, and \(\nu<30\) is a good rule of thumb for the range of \(\nu\) where the distributions differ appreciably.
Here I will assume that both the experiment-specific \(\mu_i\)s and the participant-specific \(r_{e,i}\)s have \(t\) distributions. Here is the new model:
\[ \begin{aligned} \text{Participant-level variation: }r_{i,e}^*&\sim \mathrm{Truncated\ Student\ t}(\nu,\mu_e,\sigma^2_e,(\underline r_{i,e},\overline r_{i,e}))\\ \text{Experiment-level variation: }\mu_e&\sim \mathrm{Student\ t}(\bar\nu,\bar\mu,\bar\sigma^2)\\ \log \sigma_e &\sim N(M,S^2)\\ \text{priors: } \bar\mu&\sim N(0,10)\\ \bar\sigma&\sim\mathrm{Cauchy}^+(0,10)\\ M&\sim N(0,10)\\ S&\sim \mathrm{Cauchy}^+(0,10) \end{aligned} \]
Note that I have kept the experiment-specific augmented \(\sigma_e\)s, but for simplicity I will not be allowing \(\nu\) to vary between experiments.
We need to be especially careful here when calculating \(\rho\), the fraction of the variance of \(r_{e,i}\) that is due to between-experiment variation. This is because the variance of the \(t\)-distribution is not equal to the parameter \(\sigma\). In fact, it is:
\[ V(t(\nu,\mu,\sigma))=\begin{cases} \frac{\nu}{\nu-2}\sigma^2 &\text{if } \nu>2\\ \text{undefined} & \text{otherwise} \end{cases} \]
I will therefore not comment on \(\rho\) for this estimation.
You can see this in the generated quantities
block at the bottom of this Stan program:
// 2level_interval_sd_t.stan
// a 2-level hierarchical model for aggregating risk preferences
// treating choices as revealing intervals
data {
int<lower=0> N; // number of observations
vector[N] rlb; // lower bound for r
vector[N] rub; // upper bound of r
int nexpt; // number of experiments
int exptID[N]; // experiment id
vector[2] prior_MU; // mean of r in population
real prior_sigma_expt; // sd of r at experiment level
vector[2] prior_sigma_i_M; // m and sd of log-median sigma_i
real<lower=0> prior_sigma_i_SD; // sd for sigma_i
real<lower=0> prior_nu_expt; // prior for nu at expt level
real<lower=0> prior_nu_i; // prior for nu at individual level
}
parameters {
real MU;
real<lower=0> sigma_expt;
real sigma_i_M;
real<lower=0> sigma_i_SD;
real<lower=0> nu_i;
real<lower=0> nu_expt;
// augmentted data
vector<lower=0>[nexpt]sigma_i;
vector[nexpt] mu_expt;
vector<lower=rlb,upper=rub>[N] r;
}
model {
// prior
target += normal_lpdf(MU | prior_MU[1],prior_MU[2]);
target += cauchy_lpdf(sigma_expt | 0.0,prior_sigma_expt);
target += normal_lpdf(sigma_i_M | prior_sigma_i_M[1],prior_sigma_i_M[2]);
target += cauchy_lpdf(sigma_i_SD | 0.0, prior_sigma_i_SD);
target += cauchy_lpdf(nu_i| 0.0, prior_nu_i);
target += cauchy_lpdf(nu_expt|0.0,prior_nu_expt);
// hierarchical structure
target+= student_t_lpdf(mu_expt |nu_expt, MU,sigma_expt);
target+= lognormal_lpdf(sigma_i | sigma_i_M, sigma_i_SD);
// likelihood
target += student_t_lpdf(r |nu_i, mu_expt[exptID], sigma_i[exptID]);
}
Table 15.6 shows estimates from this model. Of particular interest are the new parameters \(\bar\nu\) (nu_expt
) and \(\nu\) (nu_i
). Recall that \(\nu<30\) suggests that a \(t\) distribution departs meaningfully from a normal, and we see this for the median estimates of both \(\nu\)s (although the posterior mean estimate of \(\bar nu\) is well above 30). I would conclude from this that the normal assumption probably isn’t a good one to make with these data, both at the experiment level (i.e. \(\mu_i\) is not normally distributed), and at the participant level (i.e. \(r_{i,e}\) is not normally distributed within an experiment)
Fit_interval_sd_t<-summary(
"Code/METARET/Fit_interval_sd_t.rds" |>
readRDS()
)$summary |>
data.frame()|>
rownames_to_column(var="parameter") |>
mutate(
exptID = parse_number(parameter)
)
Fit_interval_sd_t |>
filter(is.na(exptID)) |>
dplyr::select(-exptID) |>
kbl(digits=3,caption = "Parameter estimates from the model assuming heterogeneous within-experiment standard deviations") |>
kable_classic(full_width=FALSE)
parameter | mean | se_mean | sd | X2.5. | X25. | X50. | X75. | X97.5. | n_eff | Rhat |
---|---|---|---|---|---|---|---|---|---|---|
MU | 0.535 | 0.000 | 0.024 | 0.488 | 0.519 | 0.535 | 0.552 | 0.584 | 5507.149 | 1.000 |
sigma_expt | 0.156 | 0.000 | 0.022 | 0.116 | 0.140 | 0.154 | 0.170 | 0.203 | 4285.374 | 1.000 |
sigma_i_M | -0.885 | 0.001 | 0.047 | -0.978 | -0.916 | -0.884 | -0.854 | -0.790 | 4005.442 | 0.999 |
sigma_i_SD | 0.298 | 0.001 | 0.038 | 0.231 | 0.272 | 0.296 | 0.321 | 0.383 | 3427.930 | 1.001 |
nu_i | 2.927 | 0.004 | 0.150 | 2.650 | 2.822 | 2.921 | 3.025 | 3.232 | 1623.285 | 1.000 |
nu_expt | 55.167 | 4.231 | 236.743 | 2.936 | 8.234 | 15.859 | 36.389 | 367.124 | 3131.111 | 1.000 |
lp__ | -29308.651 | 2.077 | 72.261 | -29452.428 | -29357.689 | -29306.838 | -29258.540 | -29170.925 | 1210.179 | 1.003 |
15.6 R code to estimate the models
library(tidyverse)
library(rstan)
rstan_options(auto_write = TRUE)
options(mc.cores = parallel::detectCores())
D<-"Code/METARET/Data/data.csv" |>
read.csv() |>
filter(task=="HL")|>
mutate(exptID = bibkey |> as.factor()) |>
group_by(choice) |>
mutate(
rlb = min(r),
rub = max(r)
)|>
ungroup() |>
mutate(
rlb = ifelse(choice<=1,-Inf,rlb),
rub = ifelse(choice==10,Inf,rub)
)
D$bibkey |> unique()
dStan<-list(
N = dim(D)[1],
r = D$r,
rlb =D$rlb,
rub = D$rub,
nexpt = D$exptID |> unique() |> length(),
exptID = D$exptID |> as.numeric(),
prior_MU = c(0,10),
prior_sigma_expt = 10,
prior_sigma_i = 10,
prior_sigma_i_M = c(0,10),
prior_sigma_i_SD = 10,
prior_nu_expt = 10,
prior_nu_i = 10,
nsim = 1000
)
model_interval_sd_t<-"Code/METARET/2level_interval_sd_t.stan" |>
stan_model()
Fit_interval_sd_t <- model_interval_sd_t |>
sampling(
data=dStan,seed=42,
iter = 2000
,
par = "r",include=FALSE
)
Fit_interval_sd_t |>
saveRDS(
"Code/METARET/Fit_interval_sd_t.rds"
)
model_interval_sd<-"Code/METARET/2level_interval_sd.stan" |>
stan_model()
Fit_interval_sd <- model_interval_sd |>
sampling(
data=dStan,seed=42,
iter = 2000,
par = "r",include=FALSE
)
Fit_interval_sd |>
saveRDS(
"Code/METARET/Fit_interval_sd.rds"
)
model<-"Code/METARET/2level.stan" |>
stan_model()
Fit<-model |>
sampling(data=dStan,seed=42,
iter = 2000
)
Fit |>
saveRDS("Code/METARET/Fit.rds")
model_interval<-"Code/METARET/2level_interval.stan" |>
stan_model()
Fit_interval<-model_interval |>
sampling(data=dStan,seed=42,
iter = 2000,
par = "r",include=FALSE
)
Fit_interval |>
saveRDS("Code/METARET/Fit_interval.rds")
References
I downloaded this dataset on 2024-09-12. As METARET is an ongoing project, you may get different estimates to me.↩︎